When OpenAI released ChatGPT to the world, they also unwittingly opened a Pandora's box, showing everyone the good, bad, and ugly of LLMs. While it was easy to be wowed by ChatGPT's verbose language and diverse applications, one message became familiar to all its users.
The algorithm constantly mentioned a data cut-off date, proceeding which it had no 'memory' or data. It also tended to make factually incorrect statements with confidence, dubbed 'hallucinations' leading to the shine of the new AI chatbot wearing off.
However, with the latest advances in AI, there is now a new solution to the twin problems of cut-off dates and hallucinations. Known as retrieval augmented generation or RAG, this technique aims to bridge the gap between generic AI responses and contextually enriched information.
RAG introduces a dynamic component to the traditional AI generation process. By integrating a search and retrieval system, it allows large language models to pull in the most relevant and timely data from a vast array of external sources.
This might seem like a promise come true for the AI-savvy, but the extent of RAG's powers are yet to be seen. Today, it is seen as a potential power-up to the efficiency of AI-generated content. Read on to know all you need to about RAG and how it can be used for contextual content.
Exploring Forms of Retrieval Augmented Generation Architectures
Retrieval Augmented Generation is adaptable to numerous applications, each with its unique requirements and challenges. Let's decode the forms that a RAG architecture might take:
Naive RAG: The most basic form of this technology, known as Naive RAG, involves a straightforward retrieval of information which is then fed into language models to enhance the depth of the generated content. Though effective, this approach may not always account for the nuanced understanding required in complex scenarios.
Advanced RAG: Advanced RAG takes this concept further by incorporating several advanced pre-retrieval and post-retrieval techniques to provide a better context to the LLMs. This version is particularly useful in scenarios requiring a deep understanding of context.
By understanding and implementing the right type of RAG, businesses can dramatically improve the quality and relevance of the AI-generated content.
The Advantages of Using RAG in AI Systems
The benefits of using Retrieval Augmented Generation for content creation are manifold. The three main advantages of using RAG are as follows:
Theoretically Infinite Memory: Instead of going through the expensive process of training, RAG gives the LLM data that it did not have previously. This external memory is also expandable and theoretically infinite.
More Trust in the System: Sources can be cited for any claims made in the generation, increasing the confidence in LLM’s responses.
Less Hallucination: Adherence to context and a robust RAG evolution framework has been shown to reduce hallucinations in the final response.
Apart from these, RAG also brings improvements to the overall quality of generated content, such as:
Adherence and Compliance: When compared to traditional AI-generated content, RAG-enhanced content is notably superior due to its adherence to contextual relevance and accuracy.
Customization: Using RAG allows for a higher degree of customization, enabling hyper-targeted content. For instance, a company can automatically generate localized content using RAG that is not only accurate but also tailored to the linguistic and cultural nuances of each region.
Lower Rates of Misinformation: Industries such as finance or law, where the stakes of misinformation are critically high, stand to benefit immensely from the precision and reliability of RAG-enabled content.
Keeping AI Systems Agile: RAG's ability to integrate real-time data and specific domain knowledge into content generation makes it an indispensable tool for businesses aiming to maintain a competitive edge and high standards of operational transparency.
Implementing RAG for Context-Rich Content Creation
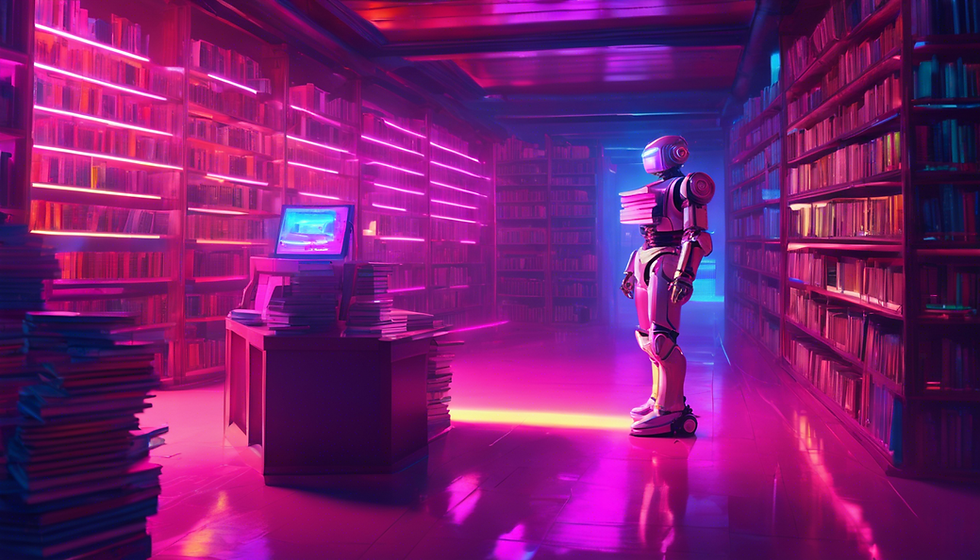
The practical application of RAG in content creation is profound. By enabling AI systems to access a diverse range of databases and document repositories, RAG empowers language models to produce not only contextually accurate but also highly personalized content.
Take, for example, a company that manufactures and services industrial construction equipment. While they might have a huge database of technical documentation for these products, it can be difficult to create content on it to demonstrate domain authority and expertise without an in-house expert.
Enter Graph RAG, an advanced iteration of the RAG approach. This version of RAG integrates a graph database to provide comprehensive contextual information to the LLM. It addresses the limitations of sending plain text chunks by offering structured information, including textual descriptions, properties, and relationships between data.
With Graph RAG, retrievers can feed the highly specific technical information to the language model, which can then in turn infuse it into the generated content. This not only demonstrates the expertise that the company holds in the space, but also creates a learning path for potential and existing customers to educate themselves on the company's offerings.
The potential of RAG in content marketing is currently untapped, but we at Yarnit have something in the works to bring this technology to our users. If you are interested in learning more about how RAG can be used for contextual content, sign up for Yarnit's upcoming webinar on how to use generative AI and data for content marketing.
Conclusion
The progression of Retrieval Augmented Generation technology marks a significant leap forward in the realm of AI-driven content creation. Its ability to merge real-time data retrieval with context-sensitive content production represents a paradigm shift from traditional methods.
If you are excited about the potential of RAG-powered AI content, stay tuned! Yarnit plans to introduce an innovative update that brings the power of RAG to your content, allowing businesses to harness the full potential of RAG in new and exciting ways.
Comments